
Abstract. Solar systems are foreseen as favorites among new energy generation technologies. Energy losses management seems relevant in this coming competitive context. On one hand, losses detection and its quantification, broken-down by each type, is necessary. On the other hand, identifying loss causes (new Machine learning and Bigdata approaches) is also needed. Contractual KPIs need to be deeper covered too, as far as the classic contractual Availability, PR and others classical indicators keep their star role. In this sense, calculation methods of real time and aggregated data of producible (possible) energy, for a better losses’ estimation is essential. Comparing Wind and PV contexts, the former enjoys better standardization in contractual KPIs than the last, whose companies, owners, EPC, O&M, and Asset Managers seems to proceed with a wide variety of contractual KPIs. In addition, contractual losses characterization uses not to be susceptible of an automatic classification, creating another need in terms of how to digitize these “manual” re-categorization, and swiftly linking them to the system, monitoring and analysis tools, or, for instance, work orders.
Introduction
Studies in collaboration with Energy International Agency, forecasted how relevant solar energy will become in next years (SolarPower Europe (2020): Global Market Outlook for Solar Power 2020-2024) arriving to Terawatts in terms of total installed power locating Photovoltaic as one of favorites generation technologies, and in general renewable energy satisfying 80% of 2030 energy demand.
For the sake of simplicity this paper will focus on grid connected systems, without local charges.
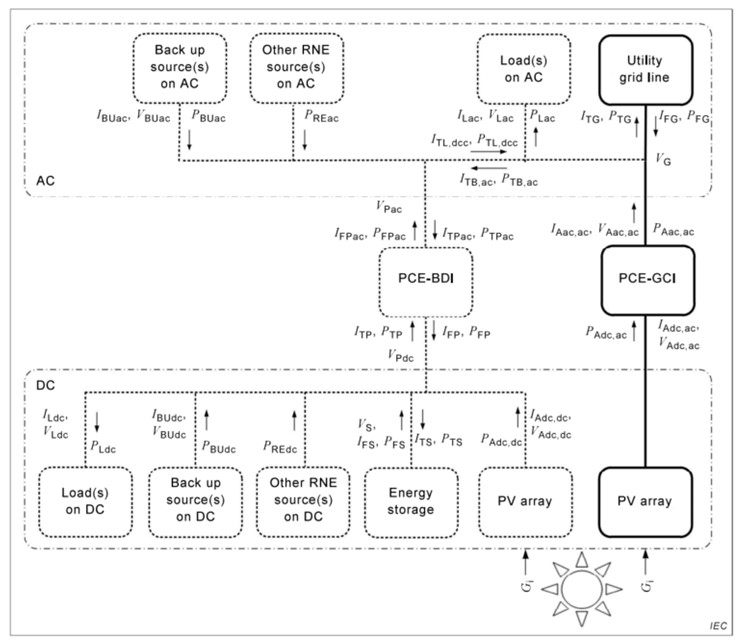
Simple empirical models are constructed to describe the performance of the PV systems under ‘normal operation” (S.K. Firth, 2010).
Losses do not tell us everything about a site, despite of getting: suitable indicators, detection, estimation even forecasting processes or early warning of failures, they are just an incomplete picture of what is happening in a PV plant. There is a huge amount of very different events with similar symptoms, due to output power from operating PV systems is highly dependent on weather and environmental conditions and consists of many interconnected units, being inevitable to encounter too many faulty situations (Mellit, 2018). However, is essential at any asset management the continuous use of proper key indicators and a suitable losses management system.
Likely causes of wrong losses estimation/detection
After two years data/conclusions gathering at ISOTROL, this list was worked out as likely causes of wrong losses estimation/detection:
-Indicators: variety related to PV losses
-Producibles: How to estimate energy you expect
-Categorization: methods of detection/classification
-Data treatment: Storing, cleaning and validation
-Centralized platform for data acquisition / supervision
-Sensorization and inputs
-Others
The first three sources will be briefly commented along the present study, and a case covered to illustrate some conclusions.
Indicators. Main contractual indicators impose a cleaning of resource signals, calculation thresholds, and to be able excluding certain events or periods, from calculations. Therefore, we will be managing operating KPIs and, on the other side, contractual ones. The former mostly related to monitoring real time data/events and the last to manage contractual liabilities, likely to demand penalties due to bad performance or guarantee management. Therefore, classical/contractual indicators are relevant in terms of benefits, leading us to identify improvement actions and thus enhance our profitability.
In terms of time-based availability, which -in general- it is measuring within a time interval the available portion of the asset DC Power, we may find in the market two main approaches when excluding external events: a) to add the excluded time as Operative time, (in numerator) or b) to deduct the excluded time from the Useful time to be considered (denominator).
In terms of energy-based availability, which -in general- it is measuring within a time interval the performance, a common practice for contractual KPIs is to add (in numerator) the external loss as an extra produced energy, so to eliminate its external effect, and achieving the contractual figure.
Another exhaustive way is to extract both from numerator and denominator (energy produced & producible) all intervals affected by any external event. If we now talk about another king of indicators, the PR, its usage has been very challenged, and many modern discussions claim PR as not enough representative as indicator, pointing other technical performance indicators as more relevant depending on the actor of the process (for instance, diffuse component, gain, tailored producible algorithms, subelements energy/time availability, etc).
Contractual PR used to be the net-adjusted-PR, i.e. the PR corrected (with temperature, module degradation and availability) plus cleaned from the impact of any external event, or sometimes, furthermore, (for the sake of a spotless operational output), even cleaned from any unavailability interval (deducting both irradiation and produced energy) or any interval with outliers.

They are in base to ordinary cases gathered along two years within a recognized engineering firm that develops PV products, projects, solutions and software for supervision and control systems: a) Ones based on PR targets derived from initial Energy Yield Assesment, b) Mathematical models c) Ones based on recent PR reference from generation unit’s empirical behavior d) Best behavior comparing the group. (See Figure 2. Expected energies). Combining these Producibles with their correction or not with factors (as, module degradation or temperature) we may obtain several scenarios. Obviously, the unavailability condition triggers will be of a main importance, and which signals will govern them, (initiating the corresponding loss calculation). However, this must be covered in a more intricated diagram which will not be in scope of this paper.
Losses Categorization. After analyzing a bulk of contractual requirements and conditions our clients have to face, in terms of losses categorization, it may be concluded their needs could be broken down into a simple types’ classification. That losses categorization could be based in answers to these questions: a) who will pay them? b) Can we control them? c) which level in the electrical cascade are they at? d) which are their likely causes? So, depending of the weight hierarchy of these questions, you will start the classification trunk tree from.
Regarding Investment performance indicators, they are mainly based in same prior KPIs but however, being separately isolated from likely causes of not reaching expected values. So, in this sense, we may find among literature the classical OPR (Operating Performance Ratio, i.e. real PR versus expected), IPR, and WPR (Investment & Weather Performance Ratio, i.e. comparing production results or Actual Irradiation versus expected ones, respectively) whose figures give owners a clue about whether their asset managers are maximizing assets or there is an improvement path yet. They are also useful to check how fair the working expected values in force are.
Producibles. For losses estimation a reference is needed. This reference or expected energy used to be called Producible Energy. Therefore Loss = Producible- Real Production. On the other hand, the unavailability condition, or trigger, will be relevant too, as you can se at the proposed diagram below. Four producible common groups have been proposed in this paper.
Examples of used categorizations of real cases will be in the scope of future studies. At the end of the day what any client/asset manager may want is an exhaustive split of energies form the very beginning (sun arriving the collector) to the last overall meter at grid boundary, plus combining in addition at every level with the complete range of possible roots of loss at that level. However, on the other hand, a simpler classification ensures a correct calculation, avoiding redundancy in calculated loss items (losses double counted). This may happen when signals & algorithms are not well modelled in more complex and exhaustive classifications. Along this study, just a case will be covered, in the zone between inverter DC input and its AC exit. This uses to comprise the common chapter of inverters underperformance, unavailability and regulation losses.
Case of losses estimation
Let`s see an example of losses estimation without too Sensorization support. Now we watch a group of elements, inverters, whose performance is needed to be controlled.
This performance is not tracked by specific sensors, and we have already obtained and split regulation and unavailability losses from it, now we may focus just on this piece of loss:
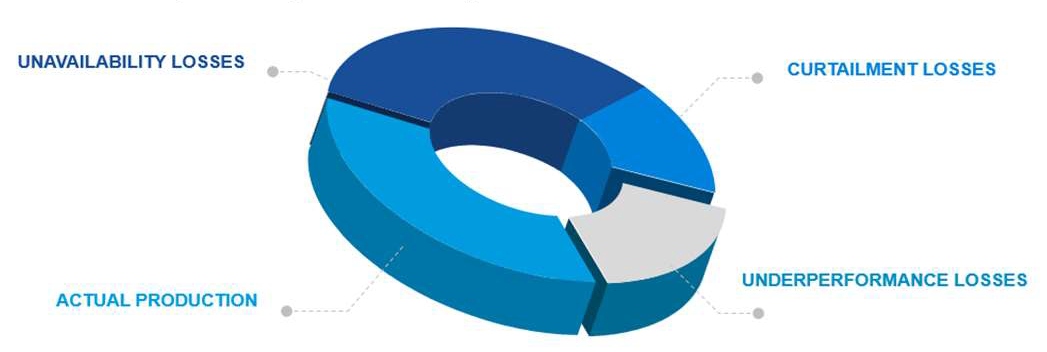
This underperformance piece of loss could be broken down into different parts: a) the inverters group low efficiency b) the datasheet/manufacturer inefficiency c) specific underperformances detectable by signals (for instance: temperature derating, clipping, etc) d) individual anomalous underperformance respect to the group.
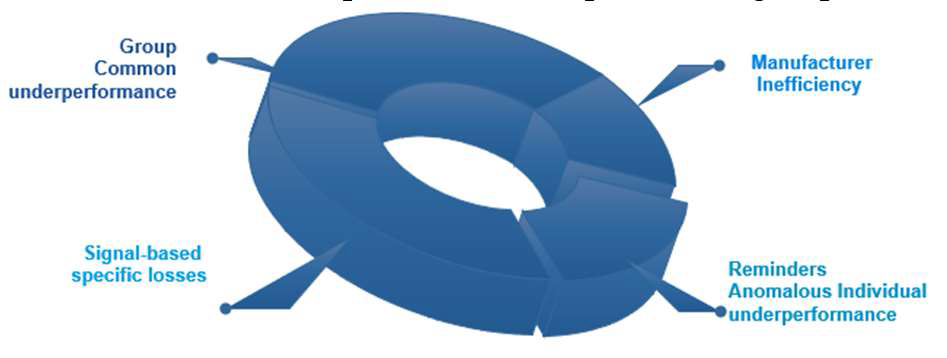
Let`s focus again in the single unknown piece among different pieces of inverters underperformance. In particular in the part corresponding to inverter individual underperformance which differentiates it from the group whose losses we want to estimate. If we call the normalized (divided by it peakPower) inverter energy as Yk = Yieldinverter_k underperformance losses can be estimated as its deviation from the group behavior (median or weighted best individual) regardless of their size:

From this bag of unexplained inefficiencies must be deducted other individuals’ losses already tracked by signals, (as mentioned above, in figure 5). Measuring distances from the inverters group behavior we can also talk about “accelerations” and to detect these accelerations, creating certain events, and to forecast based on them future issues and likely faults. All these methods can be easily applied to different electric/functional levels of elements within a PV site. Environmental challenges and energy demand growth, tempt electric utilities following ambitious strategies to ensure the optimal and secure operation of electrical power systems while utilizing renewable energy sources (K. Yurtseven, 2021). In this sense there are many studies about sensorless fault detection algorithms, which have mainly been assessed in terms of a) faults detection capability b) environmental independence c) No need of additional software d) clear implementation e) fault diagnosis capability f) operational simplicity.
Conclusions
Classical PV indicators keep an essential role in contractual scopes yet, despite not being that useful in operating or multiple plants/contracts/technologies portfolios contexts, mostly if we do not take care enough about their feeding inputs. Losses Categorization is not that easy to be standardized, unless to be complex/exhaustive and therefore leading to losses redundancy risks. Both classical PV indicators and a proper losses categorization configure a proper environment for compulsory performance analysis deducing where and how to act for achieving PV assets optimization. Sensorless fault detection by comparing individuals in a group is becoming a practical approach for losses estimation and faults early-warning and events creation.
References
6174-1, I. T. (2017). Photovoltaic system performance: Monitoring. International Electrotechnical Commission, Part 1.
6174-2, I. T. ( 2016). Photovoltaic system performance: Capacity evaluation method. International Electrotechnical Commission, Part 2.
6174-3, I. T. (2016). Photovoltaic system performance: Energy evaluation method. International Electrotechnical Commission, Part 3.
A. Chouder, S. S. (2010). Automatic supervision and fault detection of PV systems based on power losses analysis. Energy Conversion and Management 51, , 1929–1937.
A. Drews, e. A. (2007). Monitoring and remote failure detection of grid-connected PV systems based on satellite observations. Solar Energy 81, 548–564.
Europe, S. (2020). Global Market Outlook for Solar Power 2020-2024. SolarPower Europe.
K. Yurtseven, E. K. (2021). Sensorless fault detection method for photovoltaic systems through mapping the inherent characteristics of PV plant site: Simple and practical. Solar Energy 216, 96–110.
Mellit, A. T. (2018). Fault detection and diagnosis methods for photovoltaic systems: a review. Renew. Sustain. Energy Rev. 91, 1–17.
S. Silvestre, A. C. ( 2013). Automatic fault detection in grid connected PV systems. Solar Energy 94 , 119–127.
S.K. Firth, K. L. (2010). A simple model of PV system performance and its use in fault detection. Solar Energy 84 , 624–635.
W. Chine, A. M. (2014). Fault detection method for grid-connected photovoltaic plants. Renewable Energy 66, 99-110.